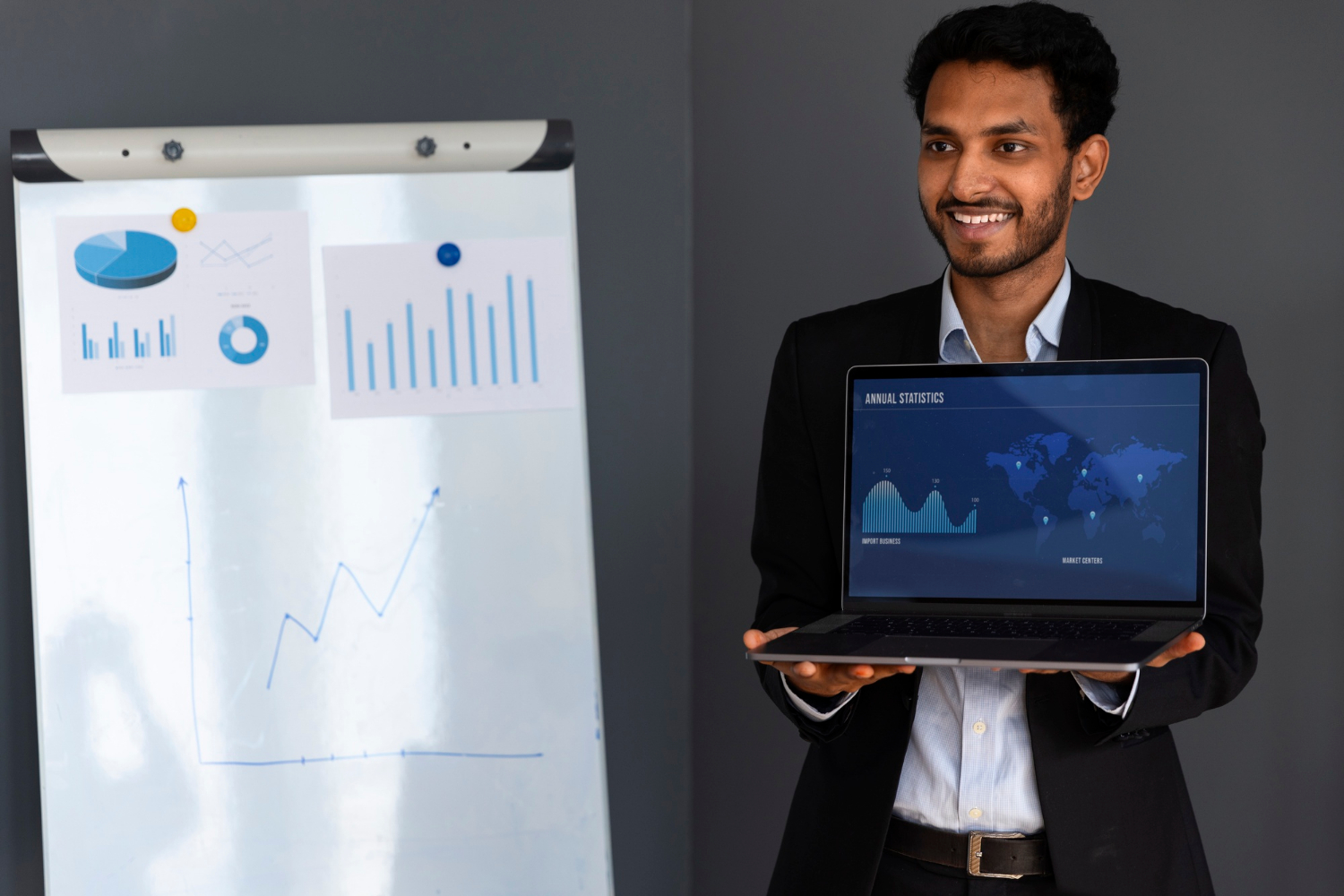
Introduction to Data Science: Analyzing the Digital Landscape
Data science is fundamentally concerned with obtaining knowledge and insights from structured and unstructured data.
Data Science’s Importance in the Digital Landscape
The importance of data science cannot be emphasised in an era dominated by digital connections. It enables organisations, researchers, and policymakers to make more informed decisions by revealing patterns, trends, and correlations in data.
Key Data Science Components
Data Gathering
Collecting appropriate data is the initial step in any data science effort. This entails gathering data from a variety of sources, such as databases, APIs, or sensor networks.
Data Preparation and Cleaning
Data is cleaned and preprocessed after it is gathered to guarantee accuracy and consistency. This stage is essential for accurate analysis and modelling.
EDA (Exploratory Data Analysis)
Data visualisation and summarization are used in EDA to find patterns and trends. This exploratory step informs the selection of appropriate models and leads subsequent investigation.
Model Construction
The next phase is to develop predictive models using clean and examined data. Machine learning techniques are used to train models that can generate accurate predictions or classifications based on data.
Model Assessment
To ensure their usefulness, models must be extensively assessed. The model’s performance is evaluated using metrics such as accuracy, precision, and recall.
Deployment
Successful models are used in real-world applications, where they might aid in decision-making or automate certain tasks.
Data Science Tools and Technologies
Programming Languages
Python and R are popular data science programming languages with substantial libraries for data processing, analysis, and visualisation.
Machine Learning Libraries
Frameworks such as TensorFlow and Scikit-Learn make it easier to develop machine learning models, making it accessible to both novice and professional data scientists.
Data Visualisation Software
Tools such as Tableau and Matplotlib assist in the creation of meaningful visualisations, which make complex data more clear and accessible.
Data Science Applications
Business Intelligence
Data science is essential in business analytics, assisting organisations in optimising processes, improving customer experiences, and making data-driven decisions.
Medical Care
Data science is revolutionising healthcare by assisting in disease prediction, patient care optimisation, and drug discovery.
Finance
Financial institutions use data science to improve efficiency and security by risk assessment, fraud detection, and algorithmic trading.
The Internet of Things
Data science is used by social media platforms to personalise user experiences, recommend content, and analyse user behaviour for targeted advertising.
Data Science Challenges
Data Security and Privacy
The growing volume of data poses privacy and security concerns. Finding a happy medium between data utilisation and data protection remains a challenge.
A scarcity of skilled professionals
The existing supply of qualified individuals in the sector is insufficient to meet the need for skilled data scientists.
Ethical Issues
As data science becomes increasingly prevalent, ethical issues such as prejudice, justice, and responsibility must be addressed.
Integration of Artificial Intelligence
The incorporation of artificial intelligence into data science processes is set to reshape how insights are generated, resulting in more efficient and accurate analysis.
Computing at the Periphery
Edge computing, which decentralises data processing, is emerging as a data science paradigm, providing faster analysis and lower latency.
Data Analysis Automation
Data scientists can focus on more difficult and strategic parts of their work when regular processes in data analysis are automated, enhancing overall productivity.
Conclusion
To summarise, data science is a dynamic field that is shaping the digital landscape. Its applications are numerous and significant, ranging from corporate analytics to healthcare and beyond. The relevance of data science in our data-driven world is only going to rise as we negotiate the hurdles and embrace future trends.